A blueprint for AI guardrails?
Jacqueline McIlroy, Sara Luck and Henry Fraser
CROSS POSTED FROM ADM+S BLOG
As Australia considers the development of ‘mandatory guardrails’ for high-risk Artificial Intelligence (AI) systems, there are interesting lessons to learn from Canada’s regulation of government use of automated decision-making (ADM). Canada’s Directive on Automated Decision-Making (Directive) dictates how Canadian government institutions should go about developing and deploying ADM. The Directive focuses on processes that encourage fairness, accountability and transparency in government decision-making, rather than prohibiting particular use cases or outcomes.
It’s a good time to assess potential approaches to AI regulation in Australia. Last year’s Royal Commission on the Robodebt scandal revealed just how harmful government ADM can be. Government agencies desperately need guidance and clarity to avoid future tragedies. In January this year, the Australian government committed to considering and consulting on legislation to introduce ‘mandatory guardrails’ for high-risk AI systems. The government appointed an Artificial Intelligence Expert Group in February 2024 to advise on these guardrails, but its mandate lasts only until June 2024. The short timeframe means that the Expert Group will probably need to build these guardrails from an existing blueprint, rather than trying to develop regulation from scratch.

Given that the government gave fairly extensive consideration to Canada’s Directive in its discussion paper on Safe and Responsible AI in Australia last year, the Directive is a strong contender. Its simplicity and clarity make it an appealing blueprint, as does its ‘risk-based’ approach that matches compliance burdens to the level of risk. It also fits the bill as a set of ‘guardrails’, although it does not use that term. So we’re going to write here about what ‘guardrails’ means (it’s not self-evident), and what the Canadian Directive reveals about the strengths and limitations of a ‘guardrails’ approach to AI regulation.
We’ll use the term ‘AI’ loosely to include data-driven and automated decision-making. Throughout its consultation process regarding AI regulation, the government has indicated that regulation of AI and ADM will be closely intertwined. Rather than getting hung up on definitions, and the fine distinctions between AI and ADM, we’ll work from the assumption that there is a lot of overlap between AI and ADM. Although the Directive only applies narrowly to government applications and ADM systems, and it does not directly regulate any other actor in the supply chain, it is nonetheless an interesting blueprint for pithy and neatly communicated ‘guardrails’. Its lessons are likely to be relevant across the supply chain of a wide range of applications of AI and ADM, and especially to government use of AI and ADM.
What are guardrails?
Guardrails could mean different things. In some quarters (among AI developers and data scientist) guardrails means technical controls built into the development process and training of an AI system. But the Australian government seems to have something broader in mind: frameworks, practices, processes, legal requirements that direct development and deployment, which go beyond technical controls. One example of guardrails might be the NSW AI Assurance Framework, a standardised process of impact assessment and documentation (and in some cases oversight) for the development and deployment of AI by the NSW government. ‘Guardrails’ evokes the image of a barrier along the edge of a road or path to stop serious falls or collisions; or perhaps of the railings at a bowling alley that beginners can use to stop balls from rolling into the gutters. Guardrails direct, protect and enable. They don’t convey the sense of a ban or prohibition, in the way that a metaphor like ‘red lines’ does.
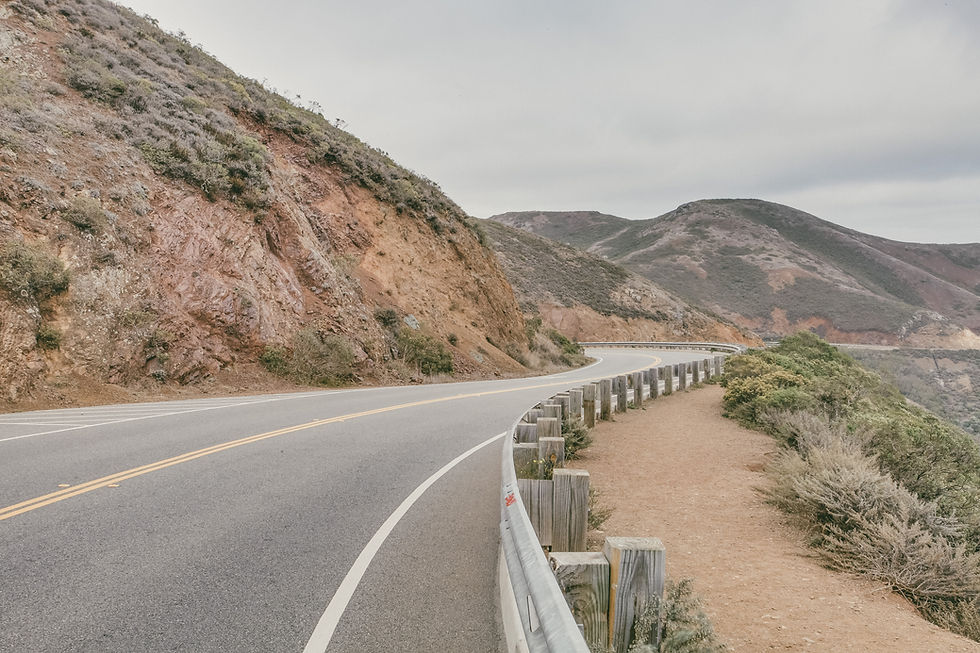
Decoding the Directive: What is it?
The Directive fits the bill as a set of ‘mandatory guardrails’. The Directive is not legislation, but it does have binding effects. It is a ‘mandatory policy instrument’ that applies to automated or partly automated decision-making by most federal government institutions in Canada. It establishes processes to guide AI development and deployment, without drawing red lines to prohibit unacceptable outcomes or uses of AI. It takes a ‘risk-based’ approach, with a set of requirements that apply in a graduated way, depending on the level of risk posed by an automated decision-making system.
The Directive classifies risks in four ‘levels’ from lowest to highest:
Level I decisions have little to no impact, with impacts that are reversible and brief.
Level II decisions have moderate impacts, with impacts that are likely reversible and short-term
Level III decisions have high impacts, and often lead to impacts that can be difficult to reverse and are ongoing
Level IV decisions will likely have very high impacts, and often lead to impacts that are irreversible and perpetual
Government institutions conduct an impact assessment, which both serves to document design and planning decisions, and to assist in working out which risk level a system falls under. The results of these assessments are public: very different to the results of applying the NSW AI Assurance framework.
One of the most appealing things about the Directive is that it is short, simple and very clear. It’s a pithy 15 pages (double spaced) — a fraction of the length of Europe’s AI Act, which runs to over 450 pages[JB1] . It deals only with government use of ADM, and is not a ‘horizontal’ regulation for AI use generally, so it doesn’t have to cover so much ground. Still, the difference in style, and commitment to brevity, is stark. The Directive’s main substance is set out in plain English in two key provisions, supplemented by appendices at the end of the document. Clause 6 covers requirements for government automated decision-making. Clause 7 deals with consequences for non-compliance. The appendices, which are the best thing about the Directive, clarify (with tables!) how each requirement applies, depending on the level of risk posed by a system. The rest is made up of legal and administrative necessaries, done about as painlessly as possible. Junior lawyers could study the Directive as an example of elegant legal drafting.
So how does it work?
Let’s have a look at some of the ‘guardrails’ in the Directive, how they might work, and what their limitations might be. The Assistant Deputy Minister of the relevant government department, or their delegate, is responsible for meeting the requirements. Failures to meet requirements may have meaningful consequences for the responsible person. We’ll illustrate by imagining how the application of these requirements might have impacted the Robodebt system. Taking a leaf out of the Directive’s book, we’ll try to do it all in a table!
Clause | Summary | How might this work in practice? |
6.1 | Algorithmic Impact Assessment The person responsible has to complete and release the results of an algorithmic impact assessment before putting the system into 'production'. The process of completing the risk assessment requires reflection on a whole range of aspects of a system including its risks and benefits, its potential impacts, the way it uses personal information, the kinds of risk management contemplated, and the structures of accountability intended to be built around the system. | Impact assessments do not by themselves mandate any particular outcome for a given system, but they do prompt reflection on key issues. This reflection happens in writing, on the record, and therefore under public scrutiny. The process may discourage government institutions from rushing into poorly conceived AI projects, and may encourage them to put greater thought into the systems. The Robodebt scandal happened because the government issued automated debt notices based on a flawed algorithm. Where welfare recipients actual fortnightly income exceeded the average fortnightly income (based on their tax return), the Robodebt system treated this as evidence of welfare overpayment, and issued debt notices, without any other evidence that welfare recipients had breached the Social Security Act. But of course, the income of people in precarious, part time or casual employment tends to fluctuate. A thorough impact assessment at an early stage might have led the government to reflect on how harmful the system could be, and to devise a more robust system. |
6.2 | Transparency The Directive imposes a range of transparency requirements including documentation of decisions about system design, release of systems’ source code for public scrutiny, provision of notice that decisions will be automated or partly automated, and mandatory plain language explanations of automated decisions to people affected by those decisions | One of the most awful things about Robodebt was the way that welfare recipients received automated debt notices from government, without any explanation. Transparency and explainability requirements like those in the Directive make the design and operation of systems like Robodebt more ‘observable’ (more easily scrutinised). This makes it easier to spot problems, easier for independent commentators like journalists and academics to investigate, and easier for affected people to challenge bad decisions. Transparency, however, does not necessarily mean that outcomes or decisions (or poor policies distilled into algorithms) will change or improve. |
6.3.1-6.3.2 | Testing and monitoring The Directive requires testing (before deployment) and monitoring (after deployment) for unintended bias and other factors that may unfairly impact outcomes. | It is stunning that no one involved in the deployment of Robodebt seemed to recognise that deviation from a welfare recipient’s average fortnightly income was not good evidence of welfare overpayment. You would like to think that more testing of the system could have created opportunities to discover this glaring error. But the thing is… there was a pilot program for Robodebt, and the Department of Human Services still went ahead with the general rollout. Testing and monitoring creates opportunities to discover problems, but doesn’t guarantee they will be found, and doesn’t require any particular response when problems are found. |
6.3.3 | Data quality This provision requires validation that data are appropriately managed, and are relevant, accurate and up to date, and are collected and used in accordance with relevant regulations and privacy law. | This is one of the few substantive requirements in the Directive. It’s not just about process, it is about the type of data you are and aren’t allowed to use for government AI. In effect it prohibits the use of unvalidated, out-of-date or inaccurate data. A requirement like this really could have made a difference to Robodebt. Other than the mean-spirited assumptions about welfare recipients underlying the program, the fundamental problem with Robodebt was the use of inappropriate, irrelevant data. At the very least, affected people could have pointed to a very clear case of regulatory non-compliance, rather than having to rely on complicated, creative legal arguments to pursue justice. |
6.3.5 | Peer review Level III and level IV systems have to be audited by independent experts, or explained in peer reviewed journal publications. | This is a unique take on algorithmic audit, an idea that has a lot of clout in commentary on responsible AI. The provision basically recognises that affected individuals might not always be best placed to detect and respond to unfair outcomes. So it creates a role for independent auditors, such as academics, experts from the National Research Council of Canada,and NGOs, to consult, and provide oversight and feedback. This is a great way of recognising the cross-disciplinary, challenging issues that government use of AI can raise. The process would be likely to flag issues that might not otherwise be detected. If the designers of Robodebt had been obliged to consult with experts from academia, civil society and beyond, they would have faced serious criticism and embarrassment at an early stage. Still, once the feedback has been received there is no strict requirement to follow it. And the option of publishing in a peer reviewed journal, instead of consulting with experts, creates the possibility of a backdoor to avoid public attention. Peer reviewed journals vary in quality, and few are widely read. Still, the peer review requirement is a pretty interesting idea that’s worth exploring and refining. |
Legal The responsible person is required to consult with the institution’s legal services from the concept stage of an automation project to ensure that the use of the automated decision system is compliant with applicable legal requirements | This provision ensures that institutions implementing ADM systems are aware of the legal issues raised by the system. This means there’s a nudge to ensure systems are legal - although government institutions are not obliged to follow legal advice, and could conceivably shop around for the advice they want. Had it applied to Robodebt, this provision would have necessitated early and ongoing consultation with legal services throughout its development and implementation in order to identify and mitigate legal risks. | |
6.3.11 | Ensuring human intervention There’s a responsibility to ensure that the automated decision-making system allows for human intervention, with a ‘human-in-the-loop’. | ‘Human-in-the-loop’ is also a much-discussed concept in the field of ‘responsible AI’. It means that a human must be involved at some stage in the decision-making process - or that affected individuals have the opportunity to appeal a decision to a human. This is an intuitively appealing idea, but there is a huge lack of certainty about how to make human oversight work at scale, and what counts as meaningful human oversight and intervention. Was there a human-in-the-loop for Robdoebt? On the one hand, debt notices were issued automatically, without requiring human approval or investigation. So you might say there was no human-in-the-loop. On the other hand, affected individuals could challenge the debts, and engage with human administrators. The problem was that the process for doing so placed totally unreasonable demands on affected individuals. So having a human somewhere ‘in the loop’ was not much help, given the other features of the system. At best, human-in-the-loop promises protection from arbitrary, cold automated decisions. At worst, it can undermine the potential benefits of automation, by slowing everything down to the speed of the human; or rubber stamp decisions that are, in practice, basically impossible to challenge. Requiring some kind of human oversight of automated decision-making systems makes sense, but working out how to implement human-in-the-loop requirements is likely to be an ongoing challenge for the Expert Group, industry and policymakers. |
6.4 | Recourse The Directive provides options for affected people to challenge government decisions that are partly or fully automated | This provision gives greater force to the transparency provisions in the Directive. If individuals can readily challenge decisions, based on clear information about AI systems, government institutions will be under greater pressure to ensure that decisions are fair. This provision does, however, rely on the initiative of individuals dealing with the government. In high- risk areas like social welfare, individuals may not be well-positioned to mount effective challenges. Again, the devil is in the detail. Strictly speaking, there were avenues for individuals to challenge Robodebt decisions. The problem was that it was just so difficult, stressful, and demanding; an additional, unreasonable burden on people who were already likely to be experiencing other forms of vulnerability. |
7 | Consequences Failures to comply with the Directive can result in consequences for responsible individuals and for institutions, with graduated severity. Penalties for individuals range from minimal and moderate consequences such as training and reassignment for minor non-compliance, to serious penalties such as demotion, loss of bonus, and termination. | This provision gives the Directive real teeth, and creates strong incentives for compliance. Let’s imagine that data quality requirements had applied to the Robodebt system, and it had fallen afoul of them. With specific provision for consequences of this kind, senior public servants could have found themselves demoted or even fired. Part of what seemed to have driven Robodebt was a willingness by public servants to go along with politicised demands from government ministers. The prospect of serious, personal penalties might have shifted the balance of incentives, and discouraged this lack of independence. |
Navigating boundaries: 'guardrails vs 'red lines'
The Directive, in steering government institutions towards fairer, more transparent and more accountable use of AI certainly meets the ‘guardrails’ brief. The Directive’s requirements, as indicated in the analysis above, work together to guide, rather than prohibit what can and can’t be done with AI. The Directive and its process-based approach have much to recommend them. The requirements are simple, and the Directive is clear and user-friendly. Though our legal systems are different, Canada and Australia share a common-law heritage, and there are many similarities in the structure of our government and public service. Australian government agencies don’t have a practice of issuing binding Directives of this kind, but a policy, a guideline, or a regulation, might achieve a similar effect.
A key point of attraction is that the Directive starts with the use of AI by government: an area where regulation is likely to be least controversial. Few would disagree, after Robodebt, that government use of AI and ADM must be more effectively managed and regulated. And, since the Directive only deals with government, it bypasses the need for a drawn-out legislative process (that may be one reason why it is such a neat and tidy document). It is telling that Canada’s draft bill on AI regulation for the private sector has faced considerable delays, and has not yet been able to pass through parliament, leaving Canada’s government to issue voluntary codes on private sector use of AI in the interim.
The other advantage of starting with rulemaking for government AI (rather than AI in general), is that it avoids difficult policy questions about balancing ‘AI safety’ against innovation. The Directive doesn’t risk chilling investment in AI because it is solely focused on government agencies. An approach based on the Directive is therefore likely to avoid pushback from business and private users of AI about restricting innovation due to ‘red tape’… at least for now.
In the meanwhile, the ‘guardrails’ for government could operate as a regulatory sandbox, permitting the government to learn from experience before regulating more broadly. Indeed, the Canadian government has reviewed and amended the Directive three times in 3 years, which shows the agility of using a policy instrument, rather than legislation, to test and iterate AI regulation. (It also showcases the Canadian government’s commitment to good regulatory practice following technological developments – something that Australia would also do well to emulate. The last public review of the Directive added the peer review mechanism described above, and references to generative AI that were accompanied by a guidance document.)
The question the Australian government, and perhaps the Expert Group on AI, will need to answer, though, is whether ‘guardrails’ are enough. Most of the Directive’s requirements rely on disclosure, explanation, oversight, testing and other similar mechanisms to achieve goals such as fairness, accountability and transparency. There is a venerable tradition of process-based regulation, and kinds of processes contemplated by the Directive are likely to create strong pressures to develop and deploy AI and automated decision-making responsibly. It is easy to imagine the Directive having a meaningful effect by creating a series of overlapping nudges.
And yet, the Robodebt Royal Commission Report showed that the government continued to pursue Robodebt, long after it was apparent that it was unfair and probably illegal. Despite the cliché, sunlight is not necessarily the best disinfectant, and nudges might not change the course of a government agency that is heavily committed to its path and motivated by cost-savings.
There is another issue. Aren’t there some uses of AI (no matter how transparent, how well-overseen, how robustly tested) that we might not, as a nation, want to accept? If the object or outcome of a system is fundamentally harmful and unfair, knowing that the system was developed transparently and with good data is cold comfort. Or to return to our ten pin bowling metaphor: it is all very well to put up ‘guardrails’ to stop gutterballs, but maybe there are some balls that should not be launched down the alley in the first place. We have to decide whether to rely on process-based regulation, or to incorporate an approach more akin to ‘product-based’ regulation, with rules about the nature of the final artefact and not only the processes used to develop it.
This is where we confront the limits of a metaphor like guardrails. Is there enough flex in the idea of mandatory ‘guardrails’ to include a concept of prohibited uses of AI? Or do we need to also bring ‘red lines’ into the conversation? Europe’s AI Act prohibits certain applications of AI, including various forms of real-time biometric identification, overbroad social-scoring, and certain kinds of manipulation. It also imposes a positive risk management requirement for ‘high-risk’ AI systems. Providers are not permitted to put these systems into use until risks have been evaluated, and reduced to an acceptable level. It imposes product-based requirements on top of process-based ones.
Of course, deciding which risks from AI are acceptable, and which are not, is incredibly challenging. It is the kind of exercise that engages deep policy questions about rights, safety, efficiency, public interests, innovation, social justice and a whole range of other issues. Until those conversations run their course, the Directive is a great blueprint for a starting point for AI regulation. Whatever its limitations, a simple, clear, process-based set of requirements for one of the highest risk uses of AI (government decision-making) would be a huge win for safe and responsible AI in Australia. But it should be the beginning, and not the end, of Australia’s journey toward effective AI governance.
Комментарии